As artificial intelligence (AI) weaves its way deeper into the fabric of our lives, from healthcare diagnoses to financial decisions, a critical question arises: can we trust these algorithms? Black-box models, while potent, leave us in the dark regarding their reasoning and decision-making processes. This lack of transparency breeds distrust and hinders the widespread adoption of AI’s transformative potential. Enter the burgeoning field of Explainable AI (XAI), poised to shine a light on the inner workings of these intelligent systems.
Why Explainability Matters
The need for XAI transcends mere intellectual curiosity. Consider these scenarios:
- A loan application is denied, leaving the applicant baffled and unable to address potential biases in the algorithm.
- A healthcare AI recommends aggressive treatment, yet the rationale behind this decision remains opaque, causing concern for both patient and doctor.
- Self-driving cars make critical decisions in split seconds, raising questions about accountability and liability in case of accidents.
Without explainability, we’re left with a chilling disconnect between humans and the increasingly powerful machines shaping our lives. XAI empowers us to:
- Debug and improve AI models: By understanding how and why they make certain decisions, we can identify and rectify biases, ensuring fair and ethical outcomes.
- Build trust and acceptance: Transparency fosters trust in AI systems, encouraging wider adoption and maximizing their societal benefits.
- Enhance collaboration between humans and AI: When humans understand AI’s reasoning, they can better collaborate with these intelligent systems, leading to superior outcomes.
Unlocking the Black Box
XAI isn’t a singular technique, but rather a toolbox of approaches to demystifying AI models. Here are some key methods:
- Feature importance analysis: Identifies which features in the data have the most significant impact on the model’s predictions.
- Local interpretability methods: Explain individual predictions by providing insights into the specific factors that influenced the outcome.
- Counterfactual explanations: Explore “what-if” scenarios to understand how alternative inputs or circumstances would have affected the model’s decision.
Real-world Applications
XAI is finding its footing in diverse domains, fostering trust and unlocking the full potential of AI. Consider these examples:
- Healthcare: XAI tools help doctors understand AI-driven diagnoses, enabling them to make informed treatment decisions in collaboration with patients.
- Finance: Banks can leverage XAI to explain loan decisions to applicants, promoting transparency and building trust.
- Criminal justice: XAI can contribute to fairer sentencing by offering insights into risk assessment algorithms used in the judicial system.
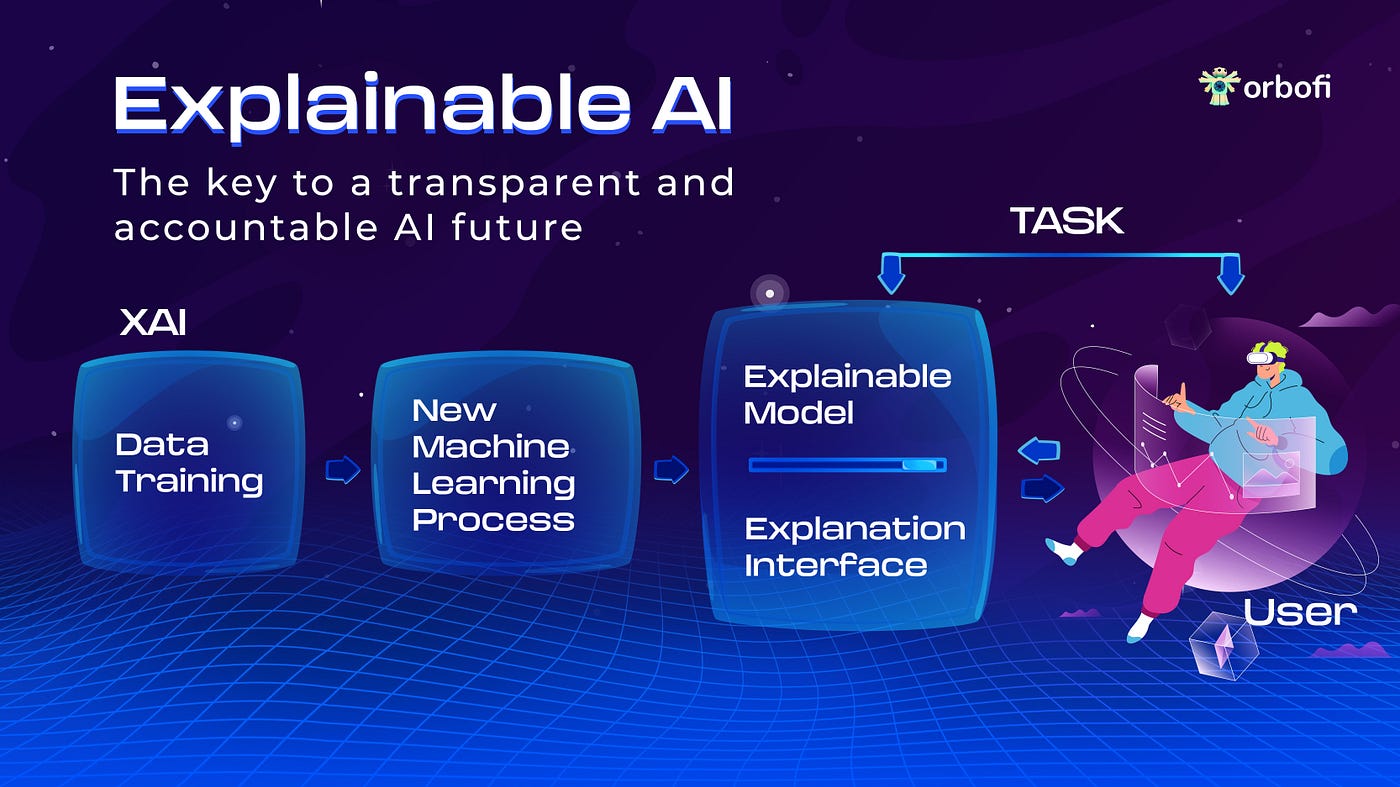
The Future of XAI
As AI continues to evolve, so too will the field of XAI. The future holds exciting possibilities:
- Development of more sophisticated explainability techniques: New methods will delve deeper into complex models, providing increasingly nuanced and granular explanations.
- Integration of XAI into AI development frameworks: Explainability will become an essential component of the AI development process, ensuring transparency from the outset.
- Wider adoption of XAI across industries: As the benefits of XAI become increasingly apparent, its use will become standard practice across various sectors.
Conclusion
The rise of XAI marks a pivotal moment in the evolution of AI. By demystifying the inner workings of intelligent systems, we can build trust, ensure fairness, and unlock AI’s true potential for good. As we move forward, let us embrace XAI as a vital tool for shaping a future where humans and machines collaborate for a brighter tomorrow.
Add Comment