For years, artificial intelligence (AI) advancements relied heavily on one crucial ingredient: human-labeled data. From meticulously annotating images for object recognition to painstakingly transcribing speech for language models, this manual effort fueled the rise of intelligent machines.
However, a new paradigm is emerging – self-supervised learning – that promises to unlock the potential of AI by training models without the need for explicit human annotations.
From Scarcity to Abundance:
The limitations of labeled data are becoming increasingly apparent. Annotating data is expensive, time-consuming, and often subjective. Moreover, the sheer volume of information available in the world dwarfs our ability to label it all. This is where self-supervised learning steps in. It leverages the abundance of unlabeled data, essentially teaching AI models to learn by “reading” and making sense of the world on their own, similar to how humans learn.
Learning from Itself:
So, how exactly does self-supervised learning work? Unlike traditional supervised learning, where models are given labeled examples (e.g., an image labeled “cat”), self-supervised learning creates its own “labels” by identifying inherent patterns and relationships within the data itself. This can be achieved through various techniques:
- Contrastive Learning: Comparing different representations of the same data to understand what information distinguishes them. Imagine showing a model two pictures of the same cat, one blurry and one clear. By learning which features remain consistent across both, the model gains a stronger understanding of what a cat “looks like”.
- Predictive Coding: Predicting missing or corrupted parts of the data. Think of a child filling in the blanks in a coloring book. By learning to anticipate what belongs where, the model develops a deeper understanding of the overall structure and relationships within the data.
- Generative Learning: Training models to generate new data similar to the input they receive. Imagine teaching a model to paint pictures like Van Gogh. By trying to replicate the style and characteristics of his work, the model learns the underlying patterns and rules that define it.
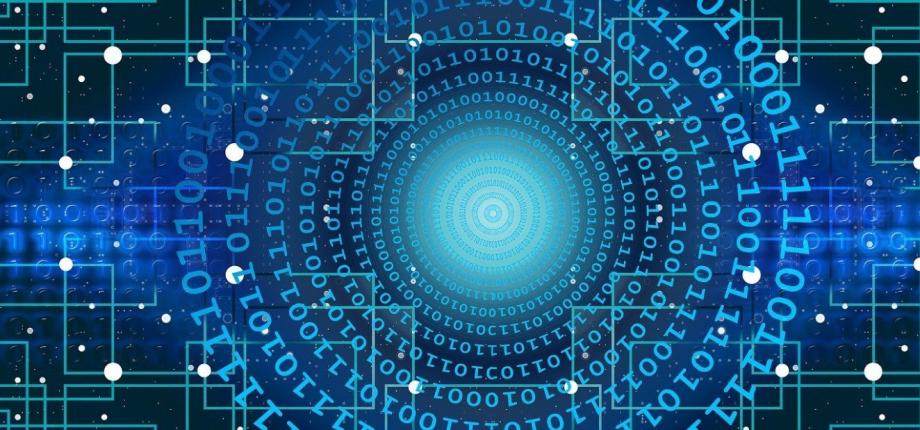
Beyond the Buzzword:
Self-supervised learning isn’t just theoretical hype. It’s already delivering impressive results in various domains:
Computer Vision:
Models trained on massive image datasets can now achieve state-of-the-art performance in tasks like object detection, image classification, and even video understanding, surpassing the accuracy of human annotators in some cases.
Natural Language Processing:
Self-supervised learning is driving breakthroughs in language models, powering tasks like text summarization, machine translation, and even creative writing, demonstrating remarkable fluency and coherence.
Reinforcement Learning:
By learning from its own successes and failures in simulated environments, self-supervised agents can achieve superhuman performance in complex games like StarCraft II, demonstrating impressive decision-making and adaptation skills.
The Road Ahead:
While self-supervised learning holds immense potential, it’s still in its early stages of development. Challenges remain, such as overcoming biases present in data and ensuring the interpretability and explainability of models trained without human-defined labels. Nevertheless, the rapid advancements in this field suggest a future where AI can access and utilize the vast ocean of unlabeled data, unlocking breakthroughs in fields as diverse as healthcare, scientific discovery, and personalized education.
The Human Element Endures:
It’s important to remember that self-supervised learning isn’t meant to replace humans entirely. Instead, it aims to democratize access to AI development and empower experts in various fields to build intelligent systems tailored to their specific needs. Human expertise will remain crucial in guiding the development and application of these models, ensuring they are utilized ethically and responsibly.
The Call to Action:
This article has provided a glimpse into the exciting world of self-supervised learning. As researchers and developers continue to push the boundaries of this technology, the possibilities seem endless. We encourage you to delve deeper into this field, explore its applications in your own domain, and contribute to shaping the future of AI by harnessing the power of unlabeled data to unlock groundbreaking solutions.
Add Comment