The COVID-19 pandemic has underscored the devastating impact of infectious diseases, reminding us of the critical need to bolster our defenses against future threats. As the dust settles, artificial intelligence (AI) has emerged as a powerful tool to transform how we prepare for and respond to outbreaks.
This article explores two high-impact applications of AI in epidemic management: supercharged simulations to predict outbreak trajectories with greater accuracy, and accelerated drug discovery pipelines to develop treatments in a fraction of the usual timeframe.
AI-Powered Simulations: Forecasting Outbreaks with Enhanced Precision
Epidemiological models have long been valuable for understanding disease spread. However, traditional models often falter with complex real-world scenarios involving vast webs of human interactions and variables. AI simulation techniques can capture nuanced transmission dynamics more effectively by:
- Analyzing massive, multidimensional datasets encompassing demographics, mobility, healthcare factors and more to gain granular insight into transmission mechanisms.
- Employing machine learning to detect subtle correlations within data that may enable more accurate targeting of at-risk groups or locations.
- Continuously updating predictions by ingesting new data in real-time to mirror unforeseen shifts in outbreak trajectories.
The accuracy improvements over traditional models are already apparent. DeepMind’s AlphaFold has predicted multiple protein structures integral for vaccine and therapy design with near-perfect accuracy. Meanwhile, DARPA’s Pandemic Prediction and Forecasting Science and Technology (PROPHET) program uses AI to generate early warnings of outbreaks by scanning news reports and social media platforms.
Case Study: Dynamic COVID-19 Models Chart Variable Trajectories
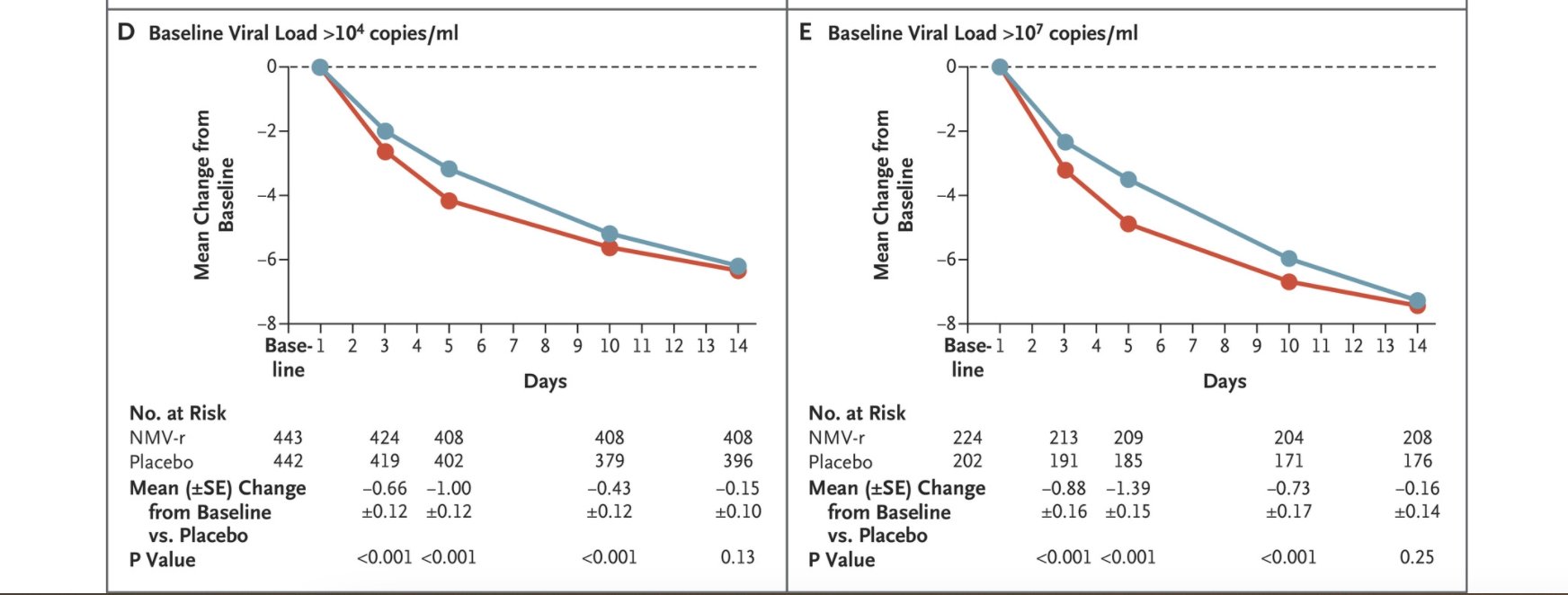
COVID-19 vividly demonstrated the limitations of static forecasting models when predicting the effects of a novel, wildly unpredictable virus. In contrast, AI-powered simulations continuously adapted projections based on emerging data on viral variants, public health interventions, vaccine uptake and more.
For instance, the Institute for Health Metrics and Evaluation’s (IHME) COVID-19 model ingests >200 parameters from multiple sources to generate weekly forecasts tailored to local conditions across states and countries. By identifying regional divergences from broader trends, this granularity enables targeted countermeasures.
The Path Ahead: Optimizing Data Sharing and Collaboration
While promising, realizing the full potential of AI epidemic tracking faces obstacles. Many health datasets remain siloed or restricted, hindering model development. Privacy concerns further limit data access. Addressing these roadblocks requires initiatives like:
- Data sharing frameworks facilitating open, ethical access to datasets for researchers.
- Industry-academia partnerships to build computational infrastructure and AI talent.
- Investment in data security and regulatory measures balancing privacy protection with modeling needs.
With thoughtful collaboration, AI could provide a live tableau of outbreak dynamics to inform life-saving decisions when next this invisible enemy strikes.
Slashing Drug Development Timelines with AI-Aided Discovery
Developing drugs and vaccines against novel pathogens has traditionally spanned years. However, with lives hanging by a thread during outbreaks, we need radical solutions to compress development timelines. AI could overhaul clumsy manual discovery pipelines by:
- High-Throughput Screening: Algorithms can rapidly screen millions of molecular candidates with therapeutic potential that would be impossible manually.
- Repurposing Existing Therapies: By matching drug databases against the viral target, AI can predict promising repurposing opportunities in days rather than months or years.
- Personalized Design: Analyzing patient-specific genetic profiles could enable tailored treatments mitigating side-effects and improving efficacy.
These techniques are no longer theoretical. AI platforms like BenevolentAI and Exscientia have shaved years off the development timeline of COVID-19 drugs like molnupiravir. Meanwhile, AI-designed cancer immunotherapies are advancing rapidly to clinical trials. As databases grow and algorithms evolve, AI could reshape the pharmaceutical playbook around outbreak readiness.
Challenges: Building Infrastructure for Integration
Effectively leveraging AI in drug discovery again faces barriers like data access and integrity issues. But more fundamentally, integrating AI within rigid research pipelines demands infrastructure revamps including:
- Modernizing data architectures from antiquated siloes towards unified systems interfacing with algorithms.
- Cultivating interdisciplinary teams combining domain and technical expertise to deploy AI responsibly.
- Incentivizing scientists to embrace AI through funding and partnerships rather than perceiving it as a threat.
Constructive dialogue, resources and visionary leadership could catalyze this culture shift.
The Next Milestone: Automating Early-Phase Discovery
Current AI interventions in drug development focus predominantly on boosting late-stage steps like clinical trials. But the biggest impacts could emerge from transforming early upstream discovery by automating iterative processes to deliver optimized molecular candidates faster.
This vision is progressing with generative chemistry techniques like reinforcement learning, adversarial networks, and transformer language models that continually refine molecular blueprints. As these tools mature, continuous drug design could beat biological threats at their perilously rapid mutation game.
Conclusion: AI to Bolster Epidemic Resilience
While not a panacea, AI’s potential to revolutionize outbreak prediction and therapeutic response heralds a new era of readiness against viral threats. But realizing this future rests upon addressing data, infrastructure and adoption hurdles through cooperation between stakeholders.
With wise investments and policy aligned towards supporting these technologies, AI could provide an invaluable line of defense against the inevitability of new epidemics emerging.
Add Comment