The rise of Artificial Intelligence (AI) is reshaping our world, and nowhere is this more evident than in the realm of autonomous decision-making. From self-driving cars to AI-powered financial algorithms, sophisticated agents are increasingly entrusted with critical choices. But who’s accountable when things go wrong? This blog tackles the crucial question: how can we prepare legal frameworks for a future where AI makes autonomous decisions?
The Challenge: From Fiction to Reality
The scenario of AI making independent decisions isn’t science fiction anymore. It’s happening now. Consider:
- Algorithmic hiring: Recruiters use AI to score job applications, raising concerns about bias and discrimination.
- Autonomous weapons: Military drones equipped with AI capabilities are being developed, sparking ethical and legal debates.
- Medical diagnosis: AI-powered tools are assisting doctors in diagnosis, but who’s liable for misdiagnosis?
These examples highlight the urgent need for a legal framework that addresses the unique challenges posed by AI decision-making.
Key Considerations for the Framework:
- Defining Autonomy: What constitutes an “autonomous” decision in the context of AI? Is it enough for an AI to process data and recommend an action, or does true autonomy require more? Legal definitions need to be clear and nuanced to avoid ambiguity.
- Liability and Responsibility: Who’s held accountable when an AI makes a harmful decision? The developer, the user, or the AI itself? Existing legal frameworks struggle to assign liability for complex algorithms, and new solutions are needed.
- Transparency and Explainability: How do we understand how AI makes decisions? Black-box algorithms, where the decision-making process is opaque, raise concerns about fairness and accountability. Explainable AI (XAI) techniques are crucial for building trust and ensuring responsible development.
- Bias and Discrimination: AI algorithms can inherit and amplify societal biases, leading to discriminatory outcomes. Legal frameworks need to address potential biases and ensure fair and equitable treatment for all.
- Human Oversight and Control: While AI may become increasingly sophisticated, some level of human oversight will likely remain necessary. Legal frameworks need to define clear lines of responsibility and control between humans and AI agents.
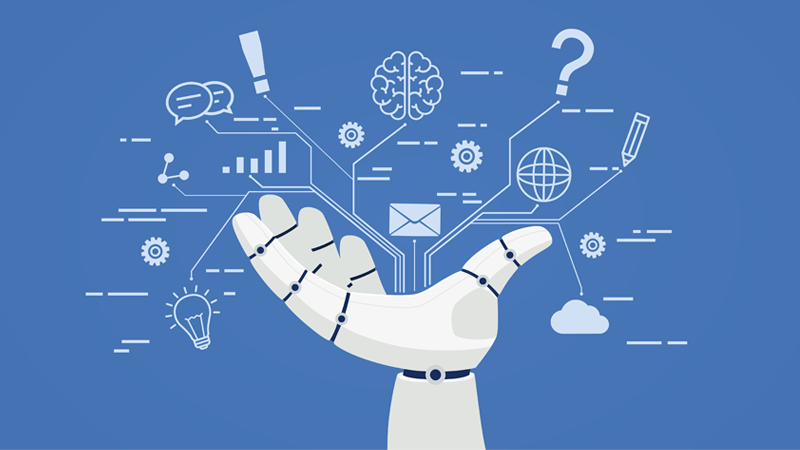
Defining Autonomous AI Decision-Making
When preparing legal frameworks, a key initial challenge lies in clearly defining what constitutes an “autonomous” AI decision-maker. There are varying levels of sophistication:
- Narrow AI focused on specific tasks
- General AI matching some human capabilities
- Superintelligence surpassing human decision-making
Most current real-world examples involve narrow AI, like algorithms making loan approval decisions. But capabilities are rapidly evolving.
For legal purposes, merely processing data and providing recommendations to humans likely doesn’t meet the threshold for autonomy. True autonomous decision-making implies the AI taking definitive actions based on its deductions. Yet even this definition raises questions.
If the AI is optimizing decisions based on initial programming by developers, how much independence does it truly have? And if advanced systems can learn and update their own decision policies, does that constitute unsupervised autonomy?
Arriving at watertight legal definitions is an ongoing challenge. But policymakers need to establish provisional taxonomies to frame the conversation productively.
Assigning Liability and Responsibility
With autonomous AI entering high-stakes domains like criminal justice and healthcare, faulty decisions carry significant ethical and legal consequences. But determining liability is far from straightforward.
If an AI diagnosis tool provides flawed medical advice leading to patient harm, who takes responsibility? The doctor, the vendor, the developer, or the AI itself? No consensus paradigm exists currently.
Human legal culpability centers on intent and negligence. But when complex neural networks make errors, intention is impossible to establish. Developers may not fully understand their creations as AI becomes increasingly inscrutable.
Some argue that liability should center on whether due care was exercised in development and deployment. Others propose making AI legally accountable as separate entities. There are no easy answers, but reframing laws to apportion responsibility more fairly is key.
Ensuring Transparency and Explainability
Closely tied to liability is the issue of transparency. Algorithmic black boxes that offer no visibility into their decision logic sharply limit legal accountability and ethical oversight.
In response, technical approaches like explainable AI (XAI) are gaining traction. XAI refers to methods for generating human-comprehensible explanations from opaque models.
Explanations can highlight influential data features behind each decision, allowing audits for bias. By peer reviewing algorithms and their assigned justifications, greater transparency is possible.
Legally requiring explainability standards would enable external testing by regulators and impacted communities. It would also force developers to better understand and improve their models through increased scrutiny.
Guidelines mandating explainable AI in defined high-risk categories could be a starting point to balance innovation with responsibility.
Countering Algorithmic Bias and Discrimination
Of growing legal concern is AI unfairness caused by biased data and models. Racist, sexist, and unethical assumptions coded inadvertently into algorithms leads to prejudicial and exclusionary effects.
Bias amplifies at scale and gets embedded into critical social structures. Discriminatory lending decisions, skewed healthcare diagnostics, uneven surveillance targeting – such harms are increasingly documented.
Some jurisdictions now recognize unfair algorithmic discrimination as a violation of existing anti-discrimination laws. Yet enforcement remains challenging.
Cross-disciplinary approaches combining law, ethics, and technology are needed to tackle this complex issue. Besides requiring explainability and external audits, diversity requirements during development could help.
Having multi-disciplinary and inclusive developer teams itself mitigates bias risks by accounting for different viewpoints. There is also scope for crafting laws focused specifically on algorithmic discrimination.
By promulgating reasonable standards of treatment based on protected attributes like race and gender, policy interventions can promote fairness.
Preserving Human Control and Oversight
A key question confronting legal frameworks is the appropriate balance of responsibility between humans and AI systems, even as autonomy increases.
While AI capabilities are projected to eventually exceed human intelligence across many domains, autonomous systems still require oversight for the foreseeable future.
No matter how sophisticated algorithms get, they lack qualities like empathy, compassion, ethics and common sense intrinsic to human decision-makers.
Laws aimed at autonomous technologies need to mandate ongoing human control, review, and direction. AI should not have unchecked power to take high-stakes actions like making arrests or issuing financial penalties.
Human oversight flowing from legal strictures provides guardrails alignment with social values. Policymakers have to take care that as autonomy advances, accountability does not diminish.
Finding the right equilibrium between automation and human supervision aligned with context remains crucial.
The Need for Global Collaboration
Because AI development and implementation increasingly transcends geographical boundaries, coordinated global action on regulation becomes necessary.
Different nations currently display varying urgency, priorities, and approaches regarding AI governance. Europe has more comprehensive AI laws focused on fundamental rights. America favors sector-specific guidelines.
China, the AI leader in areas like facial recognition, has few restrictions on technology use for social control and surveillance. Such divergence enables transgressional development inviting an ethical race to the bottom.
International alignment guided by shared democratic values and accountability measures is required. Groups like the OECD’s Global Partnership on AI promote some coordination towards this objective.
But more expansive collaboration platforms discussing technology laws and ethics are essential. AI’s cross-border reality demands cross-border governance.
The Road Ahead: A Continuous Journey
Developing legal paradigms to effectively govern autonomous AI is a complex continuous journey requiring ongoing experimentation, debate and iteration between multiple stakeholders.
Policymaking needs to be nimble, responsive and participative given AI’s rapid changes. Law should set expectations and limitations without needlessly constraining innovation and progress.
Beyond addressing immediate issues like liability and transparency, wise regulation provides the foundation for AI’s safe and socially beneficial growth. Getting governance right unlocks enormous promise for technology improving human welfare.
Through collaborative foresight and responsible development of AI, a future guided by algorithmic innovation aligned with human values becomes possible.
Add Comment