The concept of artificial general intelligence (AGI) — machines possessing human-level intelligence and reasoning abilities — has captured imaginations for decades. But while science fiction often depicts AI as fully autonomous robots, the reality underscores both remarkable progress and continuing challenges.
Breakthroughs Demonstrating Progress
The Rise of Deep Learning
Inspired by the neural networks of the human brain, deep learning algorithms have revolutionized AI capabilities. By recognizing patterns and learning from massive datasets, deep learning systems can match or surpass human performance on specialized tasks like image recognition, language translation, and strategic game play.
The victory of Google’s AlphaGo program over the world champion in the complex game of Go stunned experts and highlighted deep learning’s potential to push boundaries even in sophisticated domains. As neural networks advance, they pave the path for AI to tackle more nuanced real-world challenges.
Enhanced Reasoning and Planning
While earlier AI focused heavily on pattern recognition, modern techniques emphasize logical reasoning and planning. Symbolic AI systems construct chains of reasoning to solve problems, while probabilistic models weigh different possibilities and scenarios. This equips AI agents to handle more complex tasks involving strategy, adaptation, and decision making with incomplete information.
By combining strengths of neural networks and classical AI, systems can match human capabilities on select assignments while exceeding them on many defined tasks. Their specialized intelligence continues marching steadily toward broader competence.
The Embodiment of Intelligence
Integrating AI with physical robotics unlocks exciting real-world applications. With strengthening vision, navigation, and manipulation capabilities, robots can take on increasingly complex interactions with dynamic physical environments. Disaster response, elderly care, manufacturing, and space exploration represent just some of the promising areas for these embodied AI systems.
As algorithms better fuse perception, planning, and motor control, robots move closer to operating fluidly alongside humans in the physical world.
Enduring Obstacles and Challenges
The Generalization Challenge
Despite focused successes, many AI systems struggle to apply their learning to new situations. While humans flexibly adapt rules and knowledge to unfamiliar contexts, machines often break down dealing with scenarios beyond their specific training.
Devising techniques that support continuous learning and transferable representations remains an open research challenge limiting real-world deployment. Progress is uneven across domains, with game-playing algorithms like AlphaGo outpacing natural language interfaces in flexibility.
Interpretability Issues
The opacity of many neural networks poses barriers to trust and adoption. Unlike rule-based expert systems where humans can understand reasoning pathways, complex deep learning models operate like impenetrable black boxes.
This lack of interpretability makes it hard to diagnose failures, identify biases, and predict behavior changes. Understanding and explaining how systems reach conclusions emerges as a major priority amid growing reliance on AI.
Absent Common Sense
Despite other advances, replicating the implicit reasoning and common sense that humans acquire through lifetimes of diverse experience remains deeply challenging. AI agents often falter at puzzles and situations requiring broad real-world understanding beyond their training data.
Incorporating more foundational knowledge and strengthening common sense reasoning is critical for handling open-ended tasks across domains. This gap limits deployment potential despite focused capabilities.
Emerging Ethical Concerns
As AI systems gain competence and autonomy, ethical risks around bias, accountability, and unintended impacts grow. Critics increasingly call for deliberate efforts to ensure AI safety and avoid scenarios where advanced systems harm human well-being or erode public trust.
Research exploring alignment of AI goal systems with human values provides initial progress on this complex issue. Continued focus embedding ethics and accountability measures into AI development is critical.
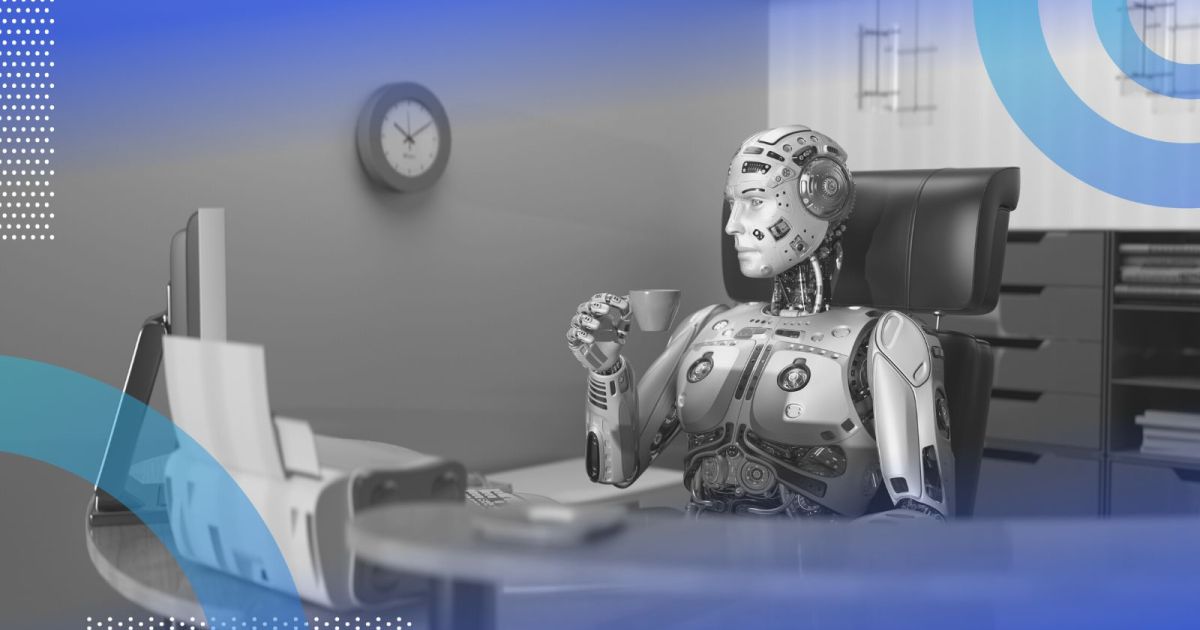
The Road Ahead: Future Directions and Possibilities
Neuromorphic Hardware
Neuromorphic computing seeks to mimic neurobiological architectures in hardware and software. This class of brain-inspired designs could overcome constraints of traditional von Neumann architectures to enable more efficient and scalable neural information processing.
With continued innovation, neuromorphic platforms offer potential to tackle pattern recognition and perception challenges more akin to biological cognition. This marks significant progress toward AI that thinks and learns like humans.
Lifelong Machine Learning
Enabling continuous learning over time is central to the quest for artificial general intelligence. Most current systems learn within fixed datasets, struggling to incorporate new knowledge and experiences to enrich their understanding.
Lifelong learning initiatives explore how to accumulate, consolidate, and transfer learned representations more flexibly across tasks. Just as humans integrate new concepts into an evolving model of the world, lifelong algorithms aim to support continual growth.
Teaming AI with Humans
Rather than replacing human intellect outright, future AI systems may excel in complementing innate human strengths. symbiotic partnerships that tighten decision-making feedback loops and augment both human and machine capabilities.
Applied thoughtfully, hybrid intelligence combining the adaptive learning and tireless precision of algorithms with uniquely human judgment, empathy, creativity, and ethics could elevate society in transformative ways.
Explainable AI
To build trust in autonomous systems influencing high-stakes decisions, explainable AI initiatives work to make opaque models more transparent. Attacking the black box problem through developing interpretable algorithms and interfaces could strengthen accountability and prevent harms.
Improved model transparency will prove essential for convincing wary adopters to deploy AI confidently across applications. This applied psychology dimension remains under-explored amid rapid technical advances.
The Ongoing Quest for Human-level AI
While science fiction visions of conscious machines remain speculative, AI systems display increasing mastery on focused cognitive tasks. Yet significant gaps endure in capability, flexibility, and transparency necessary for truly general artificial intelligence.
Overcoming these hurdles to create adaptable, trustworthy algorithms that complement humans represents the next grand challenge for the field. If navigated responsibly, this technology could usher in an era of radical productivity and human flourishing by liberating us to pursue more creative, meaningful pursuits.
Add Comment